New Diversities • Volume 23, No. 1, 2021
OPEN FORUM ARTICLE
Beyond Disciplinary Blind Spots: A Systematic Review of Research on Ethnicity and Race in Secondary Education Using Automated Text Analysis
by Lore Van Praag (University of Antwerp) and Stijn Daenekindt (Ghent University)
Abstract: Numerous scientific disciplines have shown a strong interest in studying ethnicity and race in the context of secondary education. This has resulted in a proliferation of discipline-specific perspectives on the subject, each characterized by its own blind spots. Objective. Previous reviews have applied a disciplinary approach and exclusively consider studies from one discipline. We depart from this approach and explicitly choose not to use discipline as a criterion for the studies we include. In this way, our systematic review is able to identify disciplinary blind spots and to present an interdisciplinary overview. Methodology. We study English-language articles published in Web of Science (1990-2019) on ethnicity/race/migration and secondary education/high school/comprehensive education/middle school and include all 7,620 research articles in our analysis. We analyse abstracts of these articles using automated text analysis. More specifically, we apply Topic Models to identify the core themes in the included articles. Results. We identify three clusters of topics, focusing on 1) health, 2) performance, enrolment and equity in education, and 3) psychological aspects. We discuss these clusters in depth with regard to the ways they examine the relationship between ethnicity/race and education. Conclusion. Our analysis reveals discipline-specific perspectives in the study of ethnicity/race in the context of secondary education. It also identifies gaps in the literature, within and across disciplines. In this way, the article provides researchers with insights on how they could learn from other perspectives on ethnicity/race and secondary education and stimulates interdisciplinary research.
Keywords: ethnicity; race; secondary education; Topic Modelling
1. Introduction
Over the last few decades, secondary schools have become more ethnically and racially diverse, which has generated an entirely new range of challenges and opportunities in various domains of life, such as health, education, and employment (Stevens & Dworkin, 2019). Parallel to this, there has been a growing interest in ethnic and racial diversity in educational contexts among scholars. Numerous disciplines have shown a strong interest in this subject, such as sociology (e.g., Stevens & Dworkin, 2019), psychology (e.g., Phinney et al., 2001), health sciences (e.g., Fiscella et al. 2000; Nazroo 2003), and educational studies (e.g., Jeynes, 2007). Each of these disciplines has its own research tradition with its own methodologies and loci of attention, and has therefore developed a particular approach to ethnicity/race and the educational context. This has resulted in an abundance of scattered discipline-specific perspectives on ethnicity/race within the context of secondary education. This often results in conceptual ambiguity of concepts and the lack of cross-fertilizations across fields of studies and disciplines (Klein 2000).
In this contribution, we present an overview of how disciplines differ in their perspective on the way ethnicity and race shape people’s experiences in secondary education. In this way, we offer the reader a roadmap to find her/his way in the vast and scattered literature on the subject. Moreover, this approach allows us to detect “disciplinary blind spots” on the subject. We use the term disciplinary blind spots to refer to aspects of a studied phenomenon that are not considered by a discipline. Obviously, each discipline has its own focus and may have good reasons to ignore certain aspects in its approach. Indeed, the power of each disciplinary perspective often lies in its reduction of reality by consciously ignoring certain aspects of that reality. Indeed, if this process of reduction is the result of conscious choices, this is valuable. However, it becomes problematic once certain aspects are simply not considered by researchers in a certain discipline. These forms of ‘disciplinary tunnel vision’ may hamper a more overarching understanding. A confrontation with one’s own blind spots and with other disciplines’ perspectives may help researchers to think outside the box/their disciplinary tunnel and provide them with novel insights regarding the way ethnicity and race can be defined, perceived and contextualized, and the role of ethnicity and race in the context of secondary education.
1.1. Ethnicity and Race in Secondary Education
There are numerous definitions of the concepts ‘ethnicity’ and ‘race.’ Despite the difficulties to conceptualise both concepts and the various interpretations, the most striking difference is that in most definitions the concept of ‘race’ refers to biological/physiological characteristics to distinguish groups from each other while the concept ‘ethnicity’ refers more to cultural ones (Kivisto & Croll 2012). Despite genetics not finding evidence to distinguish people based on race, the concept is still used in various contexts— in academia as well as in everyday use (Eriksen, 1993; Ladson-Billings & Tate 2006). ‘Ethnicity,’ on the other hand, is used to refer to a specific way of life, based on meanings, crucial for processes of identification and differentiation and departs from a more anthropological view (Jenkins, 2008). Both concepts continue to structure people’s lives and opportunities and their importance depends on the context in which they are used (Ladson-Billings & Tate 1995). Because of this, the concepts race and ethnicity are often (but certainly not always) entangled with migration, impacting their significance and salience (Erel, Murji & Nahaboo 2016).
The study of ethnicity and race in educational contexts has increasingly found its way into various academic disciplines, each with its own research tradition and definitions. For instance, the previously mentioned anthropological conceptions of ethnicity, which stress processes of meaning-making, differ from how other disciplines approach it. In social psychology, many scholars study ethnicity as an aspect of more generic social identity processes (Zagefka 2009). Still, in other disciplines, ethnicity is considered interchangeable with other concepts, such as race (e.g., health studies: Sheldon & Parker 1992). In this study, we aim to go beyond such disciplinary perspectives by looking at similarities and differences across disciplines.
We focus on research on ethnicity and race within the context of secondary education, as this context is deemed relevant by many disciplines. We argue that the study of ethnicity and race in secondary education is appealing for many disciplines as it is a life stage in which the effects of (early) childhood experiences become apparent. It can shape the relevance of ethnicity and race and important choices and experiences that shape young people’s future lives are made during this phase. Specifically, we address the following questions: How do different disciplines approach ethnicity and race in the context of secondary education and can we identify disciplinary blind spots? We hope that identifying these can help future studies to overcome their blind spots and, in this way, develop a more interdisciplinary perspective on the study of race and ethnicity in the context of secondary education.
1.2. Our Analytical Approach
Previous reviews depart from an explicit disciplinary approach on ethnicity/race and education. This allows them to scrutinize the subject and/or to reflect on their own discipline. For example, departing from a sociological perspective, Stevens (2007) focuses on how (ethnic/racial) inequalities in education are defined within sociology and how schools play a role in the reproduction of such unequal outcomes. Another example is the review of Busch and colleagues (2014), who study the impact of health-related behaviour and health outcomes on the actual learning of students in schools, and who use sociological theories to make sense out of the findings of the review. While this type of review studies focuses on ethnicity and race within the school context, review studies in other disciplines do not explicitly delve deeper into the educational context, but rather use education as a control or explanatory variable. This is, for instance, the case in epidemiological review studies that focus on the use of the concepts of ethnicity and race within their field of research, and discuss mainly how the effects of education on their studied outcome should be seen in a distinctive way (Comstock, Castillo & Lindsay 2004; Lin and Kelsey 2000).
The disciplinary focus that characterizes previous reviews informs their selection criteria. First, scholars restrict themselves to including only studies written within their discipline or with a disciplinary focus. This is often explicitly emphasized in the introduction of the article (e.g., Stevens & Dworkin, 2019, p. 166) or method section (e.g., exclusion of articles that were predominantly grounded in a different subject area, Lauritzen and Nodeland, 2018, 149), or is visible in the discipline-specific search terms used (e.g., obesity, body size; Amadou et al., 2013, 2; focus on Roma and education in Europe, not on travelling or tourism education, Lauritzen and Nodeland, 2018, 149). Second, some reviews exclusively study articles published in particular journals. For example, Comstock and colleagues (2004) limited their review to articles published in the American Journal of Epidemiology and the American Journal of Public Health. Third, a frequently used criteria to include articles and complete the systematic literature review is to use a snowball sampling procedure to select (additional) articles (e.g., Stevens and Dworkin 2019). This could more easily result in a biased view on a field of study or does not fully incorporate all relevant articles written on the topic.
As shown by these examples, we argue that previous reviews, while being highly valuable, may be limited in scope due to their disciplinary tunnel vision. Hence, they are unable to transcend disciplinary blind spots and to include insights from other disciplines that may enrich their research. To map the different ways in which disciplines approach ethnicity in the context of secondary education, we perform a content analysis of scientific articles. In this way, we aim to move beyond disciplinary tunnel visions on the subject by including all disciplines. We do so by applying automated content analysis on data transcending disciplinary boundaries. In this review, we analyse a large collection— over 7,000— of research articles.
Because analysing this volume of texts by hand is not feasible, we use automated content analysis. We apply Topic Models which use patterns of word co-occurrences in texts to uncover latent themes across documents (Blei, Ng & Jordan 2003). A topic consists of a set of word probabilities, and when these words are ordered in decreasing probability they closely relate to what humans would call a topic or a theme (Mohr & Bogdanov, 2013, 547). For example, a Topic Model analysis on articles in newspapers may discover a topic including the words ‘game’, ‘winner, ‘goal’, ‘injury’ and ‘competition’ with high probability, which indicates that this topic deals with ‘sports.’
Automated text analysis gives us the advantage of mapping the structure of large text data. Another advantage is that it is inductive. In this way, we minimize the change that our own disciplinary tunnel vision may affect our findings. By detecting the topics that are studied within and across disciplines, we are able to map the way disciplines differ in their approach to ethnicity and race in secondary education. In this way, we aim to reveal disciplinary blind spots. While this approach allows us to inductively map blind spots in a large collection of texts, it is content-wise not as sensitive as a close human reading. Thus, we may miss certain kinds of blind spots that are more subtle and that may remain hidden by automated text analysis. Nevertheless, our approach allows us to detect blind spots related to themes in an inductive fashion from a large collection of texts.
3. Method
3.1. Data
We focus on articles in Web of Science, the dominant academic database (Zhu and Liu, 2020). We included articles in our corpus with the following query: “TS=(ethnic* OR migr* of rac*) AND („secondary education“ OR “secondary school” OR “high school” OR “comprehensive education” OR “middle school”)”. Our application of Automated Text Analysis imposes us to limit to one language. We opted to use key terms in English as it is the lingua franca in many academic studies and journals and we reflect on the implications of this choice in the conclusion. Due to the distinct naming of educational systems and structures across countries and systems, we should remark that not all studies included in this systematic literature review are exclusively limited to secondary education. We only retain research articles, and ignore all other document types, such as book reviews or editorials. Depending on different traditions across fields of study and disciplines, this could also impact the results. We analyse the abstracts of the selected articles because they are freely available and represent a concise summary of the article; this minimizes the chance of identifying peripheral/minor topics. Our focus on abstracts, moreover, is in line with previous work (e.g., Daenekindt & Huisman 2020; Griffiths & Steyvers 2004).
This resulted in a corpus of 7,620 articles, with a total of 1,341,690 words. By selecting articles using these general keywords, we are able to apply an interdisciplinary approach and transcend disciplinary tunnel visions that characterizes previous reviews. For example, in our corpus, there are 167 different discipline labels. These labels are provided by the Web of Science database, as each journal included the Web of Science database has been assigned to one or several subject categories. All articles were published between 1990 and 2019 because abstracts are not available before 1990 in the Web of Science database. Publications before 1990 are therefore not included in our analyses. Our data show a large increase in the numbers of publications between 2010 and 2019. Although no time-related analyses will be conducted, this curve follows the recent trend in academic research in which increasingly more research is published in the form of a journal article, leading to an increase in the total amount of publications. This demonstrates that our sample of articles is in line with this trend.
Before proceeding to the analysis, we pre-processed the data. First, we lowercased all words, and we removed punctuation and numbers. In addition, we removed stop words (e.g., ‘which’, ‘and’) or irrelevant words (e.g., ‘Elsevier’) as these do not hold relevant information for our research question. We also accounted for differences between UK and US spelling (e.g., ‘behaviour’ and ‘behavior’). Next, we stemmed the data using Porter’s algorithm (Porter, 2001). Stemming reduces complexity by removing the ends of words to reduce the total number of unique words. For example, the words ‘political,’ ‘politics,’ ‘politician’ share the stem ‘polit,’ and were hence replaced with ‘polit’. Infrequently used terms were removed from the corpus as these do not contribute to understanding general patterns in the corpus. Words that appear in less than one percent of the documents were removed (e.g., Grimmer & Stewart, 2013).
3.2. Topic Modelling and Model Selection
We estimate Correlated Topic Models. The Correlated Topic Model (CTM) is an extension of Latent Dirichlet Allocation (LDA) and relaxes the assumption made by LDA that topics are uncorrelated (Blei & Lafferty, 2007; 2009). Topic models yield two sets of probabilities: the per-topic-per-word probabilities and the per-document-per-topic probabilities. The per-topic-per-word probabilities show which words are the most probable words for each topic. The per-document-per-topic probabilities show, for every document, the probability of each topic.
In line with Debortoli et al. (2016) and Fischer-Prebler et al. (2019), we used both sets of probabilities in our model selection procedure. We estimated five different models, with ten, twenty, thirty, forty, and fifty topics. We inspected these to decide on the ideal ‘level of granularity of the view into the data’ (Roberts et al., 2014, p. 1069). To evaluate which model made most sense, both authors independently investigated the most probable words for each topic and took into account the abstracts that loaded highest on the indicated topics. We selected the twenty topic model because it produced the best balance between parsimony and doing justice to the variation in the data.
4. Results
4.1. Topics and Disciplines
Before proceeding to an in-depth discussion of the topics and how they relate to each other, we first looked at how the topics related to disciplines. For this, we relied on the discipline labels provided by Web of Science. Using the standardized per-document-per-topic probabilities, we created a matrix with disciplines as rows and topics as columns. The shade of each cell relates to the probability that a topic appears in that discipline. In Figure 1, we only consider the fifteen most frequently occurring discipline-categories in our corpus to keep the figure interpretable. We discuss the content of these topics more in-depth in section 4.2. in Table 1. This heatmap shows that not every discipline covers each topic, and that there is substantial variation in the extent to which topics are used across disciplines. For example, topic seven is not clearly associated exclusively to a specific discipline as it is present in various disciplines. On the other hand, there are topics which are tightly connected to certain disciplines. For example, topic fifteen, with the highest ranked words “rural,” “migration,” “migrant,” “capital,” “labor,” “mobility,” “employments” (see Table 1), shows a very strong association with sociology. That is, this topic is almost exclusively present in sociology. This heatmap shows that research on ethnicity/race and education occurs in a wide variety of disciplines, and each of these disciplines differ in the topics they focus on.
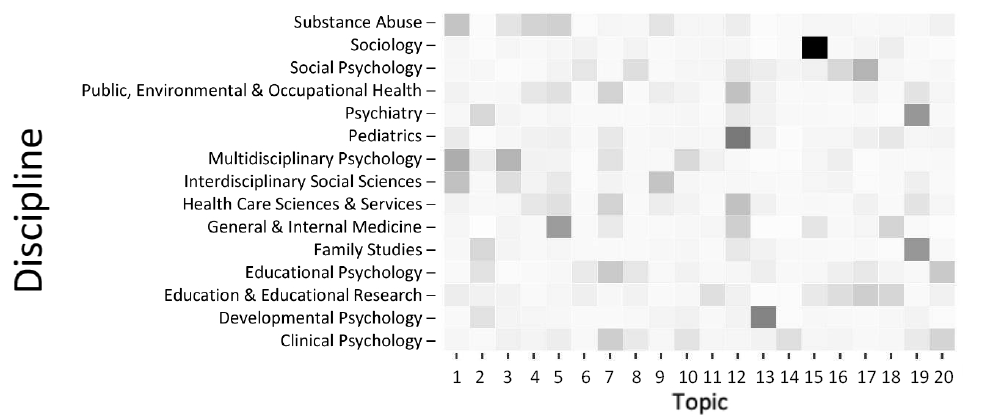
Figure 1. Heatmap Depicting the Association Between Topics And Disciplines
Table 1. The Ten Highest Ranked Words (FREX) on the Individual Level Topics
(Relative Prevalence of Each Topic Between Parentheses)
Cluster 1: Health |
Topic 9 (5.1%) alcohol, tobacco, smoking, cigarette, drinking, drug, substance |
Topic 19 (4.2%) sexual, suicide, depression, behaviors, symptoms, violence, risk |
Topic 1 (2,8%) men, health, services, women, occupational, disparities, mental |
Topic 3 (5.3%) rates, states, income, united, household, trends, mortality |
Topic 4 (5.4%) odds, weight, ratio, obesity, prevalence, pregnancy, adjusted |
Topic 5 (5.3%) patients, cancer, medical, care, clinical, screening, injury |
Cluster 2: performance, enrolment and equity in education. |
Topic 8 (3.3%) children, parents, immigrant, child, terms, family, mothers |
Topic 15 (3.3%) rural, migration, migrant, capital, labor, mobility, employment |
Topic 18 (5.8%) pupils, secondary, policy, inequality, system, power, choice |
Topic 6 (9,3%) teachers, critical, teaching, article, cultural, language, way |
Topic 11 (6.9%) college, gap, graduates, enrolled, course, attending, black |
Topic 16 (4.9%) science, career, mathematics, academic, motivation, attitudes, |
Cluster 3: Psychological aspects |
Topic 13 (5.1%) middle, bullying, victimization, aggression, peer, grade, boy |
Topic 2 (6,5%) model, predicted, mediated, relationship, stress, psychological, |
Topic 14 (4.5%) american, african, latino, discrimination, youth, identity, |
Topic 20 (4.5%) ethnic, asian, minority, groups, friends, friendships, european |
Topic 12 (3.6%) program, knowledge, intervention, randomized, recruited, skills, |
Topic 17 (5.2%) describe, new, organization, district, information, communicate, planning |
Topic 7 (5.4%) scores, scale, validity, measures, items, questionnaire, correles |
Topic 10 (3.6%) sports, athletes, physical, activity, sleep, food, intake |
We replaced stems with the most frequently occurring unstemmed word to improve legibility. |
The information of this heatmap provides the background information and exploration of the data necessary to further analyse the data. This information is necessary, as each article contains a combination of topics. Because the heatmap does not indicate which topics are combined in articles, it does not allow us to draw conclusions on disciplinary blind spots. For this, a cluster analysis is insightful as it shows us which topics tend to be combined in articles.
4.2. Cluster Analysis
We used a Q-mode cluster analysis on the document-topic probability distributions. To account for the compositional nature of the data, i.e., each row in the document-topic matrix represented sums to one, we transformed them to Aitchison composition scales and used the variation matrix to create distance measures between the topics (Aitchison 1986; Van den Boogaart & Tolosana-Delgado 2013).
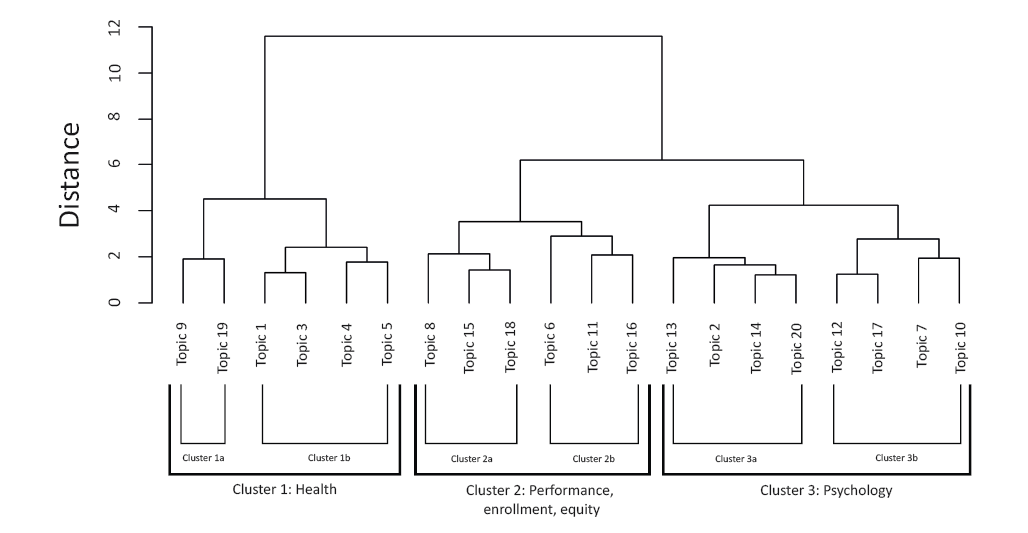
Figure 2. Dendrogram resulting from the cluster analysis on topics
Figure 2 presents the dendrogram of the cluster analysis. The distance indicates similarity between topics. Topics connected to each other at a smaller distance are more similar in terms of their distribution over the documents, compared to topics that are connected at a higher distance.
We discuss the dendrogram together with Table 1 and illustrate for each (sub)cluster our findings with exemplary articles that have the highest loading on these topics. Table 1 shows the ten highest ranked words on the individual level topics, for each of the topics, grouped according to the three main clusters that resulted from the cluster analysis.
First cluster: health.
The first group of topics (9, 19, 1, 3, 4 and 5) focus on health. For example, topic 9 relates to substance use, as is indicated by the words ‘alcohol,’ ‘tobacco,’ ‘drug.’ Topic 4 relates to research on body weight, indicated by words such as ‘weight’ and ‘obesity.’ In addition, a close reading of the abstracts loading high on this set of topics shows that these articles study patterns of health outcomes across ethnic groups. Within this cluster with a clear health focus, two larger subclusters are distinguished: the first including topics 9 and 19; and the second topics 1, 3, 4 and 5. In both subclusters the context of (secondary) education is included differently.
Cluster 1a
Articles that load high on the first subcluster of topics (topics 9 and 19) focus on lifestyle and health-related behaviour, such as substance use and risk behaviours. For example, Qiao and Bell (2016) study indigenous adolescents’ suicidal behaviours and risk factors in a nationally representative sample (Topic 19). Harrell and colleagues (2017) demonstrate how different ethnic groups in the United States use different forms of tobacco and have a different prevalence of tobacco usage (Topic 9). According to these scholars, health-related behaviours are developed during adolescence and, therefore, the context of secondary education is interesting to study in order to understand differences across ethnic/racial groups.
Cluster 1b
The second subcluster of topics (topics 1, 3, 4 and 5) relates to articles on the prevalence of health outcomes, such as obesity and mortality rates. In general, these studies focus on how health outcomes vary across a wider range of socio-demographic variables, such as gender, education and socio-economic class. The topics discussed within this cluster vary with regard to the type and specificity of health outcomes and health care behaviour. For example, Cummins and Jackson (2008) study how differences in self-assessed health varied over time (Topic 1); and Krueger and colleagues (2015) study the relationship between education and mortality in the United States (Topic 3). Other articles focus on the prevalence of particular diseases, such as the prevalence of diabetes (e.g., Borrell et al. 2009), as well as health outcomes and health care behaviour or patterns, such as the delay for presentation in cardiac care (e.g., Conigliaro et al. 2002), unequal access to health care services, like emergency department utilization (e.g., Pines & Buford 2006), or treatment effects (e.g., Kane et al. 2003), across ethnic/racial groups. In general, most studies find worse health outcomes for ethnic minority groups, and the role of education in these studies is limited to an important control variable.
Clusters 1a and 1b
Summarizing the first cluster of topics, we clearly see that articles that load high on these topics aim to explore causes of health disparities and to understand factors contributing to variation across groups. In doing so, they highlight the greater prevalence of risk factors (e.g., living in a deprived or restricted area; limited financial resources, and, important for this literature review, level of educational attainment) within ethnic minority or immigrant populations, that lie at the root of these health behaviours and outcomes. Articles examining these topics do not really depart from the discipline of educational sciences, but they include education as a context in which adolescents can be found, or as a control variable. The ways in which articles approach education, however, varies per subcluster. In cluster 1a, scholars tend to focus on a student sample, compared to studies in cluster 1b, which tend to analyse the general population. This makes sense, considering each subcluster’s focus. Given the focus of cluster 1a, namely health-related behaviour (e.g., tobacco, hookah or alcohol use), scholars consider the context of secondary education as one of the most significant places where these behaviours are more likely to occur or develop, or assume this context is associated with the period of time in which adolescents are more likely to develop such behaviours.
This contrasts with the focus of cluster 1b. When scrutinizing the relation between ethnicity and education in this subcluster, differences in educational attainment are seen as an important control factor when explaining differences in health care behaviour and outcomes. These studies frequently discuss the advantages and disadvantages of education for health-related outcomes and how it affects health care seeking behaviour and treatment. Interestingly, these studies do not necessarily find a straightforward relationship between education and health outcomes. More particularly, educational attainment increases preventive behaviour, health care practices, knowledge and resourceful networks, better living conditions, etc. (e.g., Kane et al. 2003). At the same time, higher educational attainment levels could also result in exposure to other risks associated with upward social mobility, increasing health risks and negatively impacting people’s health outcomes (e.g., Stevens et al. 1998). It is worthy of note that the benefits of educational attainment also vary across ethnic/racial groups (e.g., Borrell et al. 2009). Finally, some of these studies highlight ethnic differences in self-assessments and perceived health (e.g., Dorsey et al. 2009).
Second Cluster: Performance, Enrolment and Equity in Education
The topics in the second cluster clearly indicate a focus on educational performance, participation and inequalities. For example, Topic 8 refers to factors affecting educational performance, as it includes words such as ‘family’, ‘children’ and ‘immigration.’ Other factors relate to measures of or attitudes towards performance, such as Topic 16 which refers to key words such as ‘science,’ ‘career,’ ‘attitudes’ and ‘achievement.’ As was the case with the first cluster, two subclusters can also be distinguished in this cluster. Cluster 2a consists of topics 8, 15 and 18, and includes themes related to the family background and the home environment of students, and how these affect enrolment and achievement in education. Cluster 2b (Topics 6, 11 and 16) focuses on school-related factors and outcomes. According to the heatmap presented earlier, these topics are mainly categorized in social/educational/clinical psychology, sociology and educational research and, to a lesser extent, in pediatrics and general and internal medicine.
Cluster 2a
Articles that have many topics associated with cluster 2a concentrate on the impact of migration or belonging to an ethnic minority group on educational achievement and enrolment. In doing so, many family background characteristics are examined in order to better understand how migration or belonging to an ethnic minority group matters for educational outcomes (Topic 8: e.g., Wojtkiewicz & Donato 1995), who migrates for education (Topic 15: e.g., Pais, de Mattos & Teixeira 2018), and how this is facilitated by existing educational structures and policies (Topic 18: e.g., McGuinn 2016). In particular, considerable attention is given to family background characteristics (e.g., Raley et al. 2005) and the financial, social and cultural resources these families have at their disposal to support their children to be successful in education and realize their (relatively high) aspirations. This is illustrated by Spera, Wentzel and Matto (2009), who study parental aspirations for their children’s educational careers and contrast these aspirations against their children’s actual educational performances.
Cluster 2b
In the second subcluster, studies focus on school-related factors that explain ethnic differences in education, such as educational practices, culture and language used (Topic 6), and how they affect school/course choice, attendance and enrolment (Topic 11) and motivations and school attitudes (Topic 16). By questioning these dominant views and practices that are taken for granted within educational policies and practices, these studies apply a critical (race) perspective, which contrasts with all other clusters discussed in this article (Topic 6). For example, articles focus on how teaching practices are hard to change as they are embedded in the curricula of schools, shaped by the dominant cultural groups (Chan, 2006), or on how youngsters themselves define ‘race’ (Roberts et al. 2008). The other studies in cluster 2b further discuss how the structural disadvantage of ethnic minority groups and particular racial groups in society and education continues to reflect in their attitudes towards schooling and actual educational behaviour and performance. They do so by looking from a sociological perspective at school choices and factors influencing enrolment in higher education, and how they are organized by the opportunities these groups have (Topic 11). For example, Garcia (2006) studies how school choice decisions result in more racially segregated schools, impacting their future educational careers. More social psychological research discusses the attitude-achievement gap in general, and by doing so, these scholars also include or focus on ethnicity and/or race (Topic 16).
Clusters 2a and 2b
To summarize the second cluster, most studies focus on a wide range of factors that help to understand how ethnic/racial inequalities are maintained through education. These studies are situated within social science research. Articles with high scores on cluster 2a examine the development of human capital for migrant and ethnic minority groups, how resources within (family) networks contribute to this, and how much these factors play a role in how students perform and navigate within a particular educational system. Consequently, in the majority of these studies, parents’ educational level is included (e.g., Spera et al. 2009). Remarkably, in cluster 2a, the focus on individual level characteristics and educational policies unravels the way (ethnic) inequalities are produced and reproduced through educational policies and across (migrant) families, without considering what happens in the school context. In cluster 2b, this research is complemented by studies that examine school-related factors and school dynamics within the wider societal context. Thus, studies with high scores on the second cluster concentrate on how existing structures and policies, as well as youngsters’ family background, further impact the actual educational behaviour and outcomes of students. These studies do so by focusing on the impact policies, structures and family background have on people’s minority position they have in society and culture, the resources they have access to, and the impact on their motivations and attitudes. These studies contribute to insights into how ethnic inequalities are produced and reproduced. This is also a major topic in the fields of sociology, psychology and educational sciences. These studies apply a wider variety of research methods, compared to the first cluster. Especially studies using a critical, sociological approach (Topic 8) applied more often qualitative research methods, although the majority of the methods used in these studies remain quantitative in nature.
Third Cluster: Psychological Aspects
The third cluster of topics applies a more psychological approach to ethnicity in the context of secondary education. This is clearly visible in the heatmap, in which all topics are categorized into one or more subdisciplines of psychology, in some cases combined with other disciplines. Most articles of Topic 13 fall into the discipline of developmental psychology; Topic 14 fits into clinical psychology; and Topic 17 mainly is categorized into educational psychology. Other topics are more combined with other related disciplines, such as Topic 12 which loads high on social psychology, pediatrics, health care sciences and services, and public, environmental and occupational health. Similar to the other two main clusters, we note a subdivision of topics into two subclusters. The first subcluster 3a contains topics 13, 2, 4 and 20; the second one, cluster 3b, includes topics 12, 17, 7 and 10.
Cluster 3a
The articles in cluster 3a focus on aspects of students’ psychological development. Each topic has a particular approach, but all are related to adolescence or school life. Attention has been given to how families and students deal with psychological problems (Topic 2, e.g., Flouri & Panourgi 2014), school climate, peer processes related to victimization (e.g., Topic 13, e.g., Shirley & Cornell 2012), the development of racial identities and its impact on health and educational outcomes (Topic 14, e.g., Hurd et al. 2013), and ethnic diversity in schools and networks (Topic 20, e.g., Munniksma et al. 2017). Topics in cluster 3a vary slightly in the extent to which they concentrate on particular ethnic groups and the psychological issues with which they are confronted. Most of the psychological issues discussed in the articles that are associated with this subcluster are explicitly related to being part of an ethnic minority group or race, or having a migrant background (e.g., acculturative stress, e.g., Kim et al. 2014; school punishments, Shirley & Connell 2012; racial identity, e.g., Hurd et al. 2013). The focus on occupying a minority position in society, or belonging to a specific ethnic/racial group, is not always explicitly emphasized or the particular focus of the studies. Nevertheless, most of these studies do recognize its importance and control for it in the analyses (e.g., Flouri & Panourgi 2014). The school context and dynamics within schools take up a central role in the design of studies and theories used that score high on topics in this subcluster.
Cluster 3b
In the second subcluster, relatively more interlinkages are made to other disciplines than psychology, such as health care sciences or public health, than in cluster 3a. For instance, in Topic 12, studies focus on the evaluation of programs and interventions directed at changing health-related behaviour, both specifically related to adolescence and peer pressure, such as substance use (Shetgiri et al. 2011) and more generic health-related behaviour, like wearing glasses (Yi et al. 2015). Furthermore, research articles focus more on intervention programs, governance, and policies (especially Topics 12 and 17) compared to cluster 3a. Most of these studies and programs are explicitly directed at specific ethnic/racial groups and aim to reduce their vulnerable and disadvantaged position in society. Moreover, we note that these policies are clearly linked to ethnic diversity and the so-called integration of ethnic minority groups or migrants into society and schools (e.g., Topic 17; Bradbury 2008). Within cluster 3b, schools are seen as a governance tool to realize or promote a successful integration of ethnic/racial minority groups within the dominant or immigrant society and reduce ethnic/racial inequalities or unequal opportunities in society in general. In these articles, ethnic and racial diversity are often seen as a problem that need to be resolved, or as a potential that have been insufficiently realized or that have not yet led to positive outcomes. Furthermore, many of these articles refer to ethnic/racial segregation in society and aim to evaluate/study/implement policies which are framed into wider societal objectives and policies (e.g., Rasmussen, 2017). Finally, in order to be able to understand and evaluate such policies and programs, as well as the role ethnicity and race plays herein, research articles that study ethnic differences with regard to particular psychological or health-related outcomes are closely related, as well (namely, Topic 7 and 10). One topic in particular, Topic 7, is concerned with how these psychological constructs are measured and whether these scales are valid for particular ethnic groups or vary across ethnic groups, such as self-concept scores (Worrell, Watkins & Hall 2008) or the Reynolds adolescent depression scale (Walker et al. 2005). Examining the validity and reliability of these psychological measurements is relevant when discussing and examining ethnic differences in physical, sexual, dietary and other activities of adolescents, as is the case for studies in Topic 10 (e.g., Butt et al. 2010; Sheng & Gao 2012). The finding that there are clear differences across ethnic and racial groups inspires developing new policies or adjusting existing ones.
Clusters 3a and 3b
Articles in the third and final cluster have a clear focus on psychological outcomes. Due to the major changes and developments in adolescence – which is often a specific topic of research in psychology – the educational context plays a central role in most of these studies. While in cluster 3a more attention is given to students’ psychological development itself, research articles in cluster 3b focus more on how this differs across ethnic groups and/or is measured differently, and consequently relates to interventions and policies.
5. Discussion
When examining these clusters, some blind spots can be identified that could broaden and innovate our views on the relationship between race/ethnicity and education, both within and across disciplines. We will discuss the blind spots per cluster. First of all, the first cluster, which focuses on health, shows that a large number of studies focusing on ethnicity/race and education in our search do not really pay attention to educational outcomes, but rather focus on health outcomes, such as health care behaviours, substance abuse and health disparities. The abundance of research on these topics seems to suggest the need to look from a more holistic perspective at the school careers of youngsters and how it affects their entire lives. Especially in secondary education, ethnic and racial differences in peer influences and deviant behaviour could play an enormous role in the further development of these young people’s school careers. Furthermore, it is surprising that these studies hardly consider the wider school and societal context to interpret their results. For example, studies that load high on topics from the first cluster take the school context for granted (first subcluster) or are not even limited to school samples and merely focus on general population trends, controlling for educational background (second subcluster). These studies depart from health care sciences and substance use (cf. heatmap), and hardly discuss the relationship between health-related outcomes, education and ethnicity. More research that focuses on the processes behind this relationship that pays attention to the underlying dynamics is crucial to understand the mixed effects of education on health-related outcomes.
The second cluster contains studies focus on performance, enrolment and equity in education, and depart from the social sciences. In this cluster, factors related to migration and/or being part of an ethnic minority are considered, examining both the family environment as school-related factors. The main focus of these studies is on how these factors relate to the educational careers and outcomes of particular groups of students with a migration background or belonging to ethnic minority groups. In line with currents of thought within social science disciplines, the educational context is often an integral aspect of the research question and focus. Different aspects of this educational context, such as achievement outcomes, enrolment policies or existing structures within the studied educational systems are considered and critically assessed. At the same time, attention has been paid to the ways in which migration history or ethnic minority position matters within this context. These research trends are, for instance, more in line with intra-disciplinary systematic literature reviews that delineate research traditions focusing on ‘racism and discrimination’ or ‘motivation and attitudes towards school’ (e.g., Stevens & Dworkin 2019). As these studies are very much concentrated on the educational and migration/minority context in which adolescents find themselves, they do not fully capture other interfering/supporting factors, such as substance abuse, or the relevance of these factors in the lives of adolescents. The relevance of these life domains compared to each other has not yet been not yet explored as they are not studied together. Furthermore, these studies focus on how having a migration background or belonging to an ethnic minority shapes students’ educational careers, but do not surpass this life phase as they do not delve deeper into their consequences for students’ future lives.
Studies in the third cluster focus exclusively on how adolescents experience (school) life, and how programs, policies or other interventions can be developed to improve this experience. These studies incorporate the educational structures in which adolescents find themselves, but focus on the psychological issues ethnic minorities or migrants are dealing with, and how this relates to the overall position of particular ethnic groups in society. Schools are, rather, seen as governance tools to compensate for these structural positions; they are regarded as crucial institutions in society to promote change or to promote the successful integration of ethnic minorities and migrants in society. Overall, studies in the third cluster can be clearly be categorized with the discipline psychology, applying a very (narrow) intra-disciplinary focus, or study programs, policies and other ways to deal with such issues. This focus gives a clear picture on how being part of minority groups matters overall in life, but tends to neglect how institutions are structured by society.
6. Conclusion
The present study shows that the combination of ethnicity/race and secondary education is studied in a wide variety of disciplines, ranging from health sciences, to sociology, clinical psychology and educational research. Moreover, the findings of this literature review go beyond disciplinary tunnel visions. This study allows us to further identify other relevant fields, concepts and studies, related to your central concepts, and reflect upon how they are studied in other geographical regions as well. This is frequently missed in prevailing academic canons that mainly draw upon research within the same (sub)discipline and field of study. Departing from a broader scope, using automated text analysis, is particularly valuable when delineating a research domain and/or question and to enlarge one’s literature review, which further helps to avoid the pitfalls of one’s specific (sub)discipline. Furthermore, by revealing disciplinary blind spots on the subject, this overview helps to make researchers’ implicit reasons to include education and ethnicity in their study more explicit, and contributes to further interdisciplinary theory-building and/or more critically reflecting on the premises, canons and traditions in one‘s own discipline.
In our systematic literature review, we identified three clear clusters, each characterized by a distinct approach on education and ethnicity. Each cluster studies one aspect of the encompassing and structuring impact of both education and ethnicity on all kinds of life opportunities and chances. It should be noted that some ethnic/racial/migrant groups are more frequently studied with regard to a particular topic. For instance, ethnic minorities and migrant children are more represented in Topic 8; migrant populations are more studied in Topic 15; and all groups are included in Topic 18. Also these topics demonstrate that ‘ethnicity’ and ‘migration’ are more often studied together when discussing issues such as belonging in education (e.g., cluster 2a) or ‘race’ and ‘structural discrimination’ (e.g., cluster 2b). Similar nuances are noted with regard to the educational level examined. This bias with regard to studied ethnic or migrant groups related to specific topics could also relate to the needs these groups have and the distinct positions they have across societies.
Some limitations of this paper need to be noted. First, the disciplines included in our study aligned with the pre-existing categorizations found on the Web of Science database . The technique to develop the heatmap also did not allow us to consider all disciplines. Second, due to our selection of journals and papers in Web of Science, the search terms in English we used, as well as our choice to include ‘race’ in the search terms for this study, there is a clear limitation with regard to language in which papers are published, and consequently, a geographic bias of the selected and discussed articles. This selection bias reflects the existing power dynamics in the academics and beyond. As most highly ranked journals, included in Web of Science, publish in the United States of America and the United Kingdom, and most journals are published in English (Mongeon & Paul-Hus 2016). For this reason, journal articles conducted within these countries weigh more heavily in the current overview as well. This also has consequences for the ways in which ethnic and racial inequalities in education are approached in research. This may be particularly relevant because the concepts ‘race’ and ‘ethnicity’ have very contextualised meanings and historical roots in each country (Ladson-Billings & Tate 1993; Jenkins 1993).
Departing from this interdisciplinary systematic overview, future research could focus more on specific clusters and/or delve deeper into the research traditions of each cluster by combining automated text analysis with qualitative review techniques. More generally, we hope that our article contributes to further interdisciplinary work by helping researchers to go beyond their disciplinary blind spots and to develop new innovative ways in which education and ethnicity could be studied.
7. Acknowledgements
We would like to thank the reviewers and Jef Vlegels for their insightful comments and suggestions. We also want to thank Marie Van Praag for her support in visualizing Figure 2.
Note on the Authors
Lore Van Praag (MA, PhD Sociology, Ghent University) is the head of the Centre for Migration and Intercultural Studies at the University of Antwerp. Her research interests are educational success and ethnic inequalities in schools, tracking, discrimination, early school leaving, and ethnography, environmental change and migration.
Email: Lore.VanPraag@UAntwerpen.be
Stijn Daenekindt holds an MSc in Statistics (2012, KU Leuven) and a PhD in Sociology (2015, Ghent University). Most of his work is situated in the fields of cultural sociology, education research, and political science. Currently he works at Ghent University where he is affiliated with the Centre for Higher Education Governance Ghent (CHEGG) as a postdoctoral researcher.
Email: Stijn.Daenekindt@UGent.be
References
AITCHISON, J. 1986. The statistical analysis of compositional data. Monographs on statistics and applied probability. London: Chapman & Hall.
AMADOU, A., P. Ferrari, R. Muwonge, A. Moskal, C. Biessy, I. Romieu, and P. Hainaut. 2013. “Overweight, obesity and risk of premenopausal breast cancer according to ethnicity: a systematic review and dose‐response meta‐analysis”. Obesity Reviews 14(8): 665-678.
BAE, S., R. Ye, S. Chen, P. A. Rivers, and K.P. Singh. 2005. “Risky behaviors and factors associated with suicide attempt in adolescents”. Archives of Suicide Research. 9(2):193-202.
BLEI, D. M., A.Y. Ng, and M.I. Jordan. 2003. “Atent dirichlet allocation”. Journal of Machine Learning Research 3:993-1022.
BLEI, D. M., and J.D. Lafferty. 2007. “A correlated topic model of science”. The Annals of Applied Statistics 1(1):17-35.
BLEI, D. M., and J.D. Lafferty. 2009. “Topic models”. In: A.N. Srivastava and M. Sahami, eds., Text Mining: Classification, Clustering, and Applications. New York: Chapman and Hall/CR.
BRADBURY, M. D. 2008. “Parents involved in community schools v. Seattle School District No. 1: Dubious prospects for diversity as a compelling governmental interest”. Review of Public Personnel Administration 28(4):385-391.
BRADBY, H. 2003. “Describing ethnicity in health research”. Ethnicity and Health 8(1):5-13.
BROWN, J. S., S.O. Meadows., and G.H. Elder Jr., 2007. “Race-ethnic inequality and psychological distress: Depressive symptoms from adolescence to young adulthood”. Developmental Psychology. 43(6):1295.
BOURDIEU, P. 1988. Homo Academicus. Stanford: Stanford University Press.
BORRELL, L. N., N.D. Crawford, F.J. Dallo, and M.C. Baquero. 2009. “Self-reported diabetes in Hispanic subgroup, non-Hispanic black, and non-Hispanic white populations: National Health Interview Survey, 1997-2005”. Public Health Reports 124(5):702-710.
BUSCH, V., A. Loyen, M. Lodder, A.J. Schrijvers, T.A. van Yperen, and J.R. de Leeuw. 2014. “The effects of adolescent health-related behavior on academic performance: a systematic review of the longitudinal evidence”. Review of Educational Research 84(2):245-274.
BUTT, J., R.S. Weinberg, J.D. Breckon, and R.P. Claytor. 2011. “Adolescent physical activity participation and motivational determinants across gender, age, and race”. Journal of Physical Activity and Health 8(8):1074-1083.
CHAN, E. 2006. “Teacher experiences of culture in the curriculum”. Journal of Curriculum Studies 38(2):161-176.
COMSTOCK, R. D., E.M. Castillo, and S.P. Lindsay. 2004. “Four-year review of the use of race and ethnicity in epidemiologic and public health research”. American Journal of Epidemiology 159(6):611-619.
CONIGLIARO, J., J. Whittle, C.B. Good, M. Skanderson, M. Kelley, and K. Goldberg. 2002. “Delay in presentation for cardiac care by race, age, and site of care”. Medical Care 40(1):1-97.
CRISP, G., A. Taggart, A. and A. 2015. Nora. “Undergraduate Latina/o Students: A Systematic Review of Research Identifying Factors Contributing to Academic Success Outcomes”. Review of Educational Research 85(2):249-274.
CUMMINGS, J. L., and P. Braboy Jackson. 2008. “Race, gender, and SES disparities in self-assessed health, 1974-2004”. Research on Aging 30(2):137-167.
DAENEKINDT, S., and J. Huisman. 2020. “Mapping the scattered field of research on higher education. A correlated topic model of 17,000 articles, 1991-2018”. Higher Education 80:571-587.
DAI, H., and J. Hao. 2017. “Electronic cigarette and marijuana use among youth in the United States”. Addictive Behaviors 66:48-54.
DEBORTOLI, S., O. Müller, I.A. Junglas, and J. vom Brocke. 2016. “Text mining for information systems researchers: an annotated topic modeling tutorial”. CAIS.39:7.
DORSEY, R. R., M.S. Eberhardt, and C.L. Ogden. 2009. “Racial/ethnic differences in weight perception”. Obesity 17(4):790-795.
EREL, U., K. Murji, & Z. Nahaboo. 2016. “Understanding the contemporary race-migration nexus”. Ethnic and Racial Studies 39(8), 1339-1360.
ERIKSEN, T.H. 1993. Ethnicity and nationalism. Anthropological perspectives. London: Pluto
Press.
FISCELLA, K., P. Franks, M.R. Gold, and C.M. Clancy. 2000. “Inequality in quality: addressing socioeconomic, racial, and ethnic disparities in health care”. Jama 283(19),2579-2584.
FISCHER-PREßLER, D., C. Schwemmer, and K. Fischbach. 2019. “Collective sense-making in times of crisis: Connecting terror management theory with twitter reactions to the Berlin terrorist attack”. Computers in Human Behavior 100:138-151.
GRIFFITHS, T. L., and M. Steyvers. 2004. “Finding scientific topics. Proceedings of the National Academy of Sciences”. 101(1):5228-5235.
GRIMMER, J., and B.M. Stewart. 2013. “Text as data: The promise and pitfalls of automatic content analysis methods for political texts”. Political Analysis 21(3):267-297.
GROSS, C., A. Gottburgsen, and A. Phoenix. 2016. “Education Systems and Intersectionality”. In: A. Hadjar and C. Gross, eds., Education Systems and Inequalities: International Comparisons, Bristol: Bristol University Press.
HARRELL, P. T., S.M.H. Naqvi, A.D. Plunk, M. Ji, and S.S. Martins. 2017. “Patterns of youth tobacco and polytobacco usage: The shift to alternative tobacco products”. The American Journal of Drug and Alcohol Abuse 43(6):694-702.
HURD, N. M., R.M. Sellers, C.D. Cogburn, S.T. Butler-Barnes, and M.A. Zimmerman. 2013. “Racial identity and depressive symptoms among Black emerging adults: The moderating effects of neighborhood racial composition”. Developmental Psychology 49(5):938.
JENKINS, R. 2008. Rethinking ethnicity. Arguments and explorations. Los Angeles: SAGE.
JEYNES, W. H. 2007. “The relationship between parental involvement and urban secondary school student academic achievement: A meta-analysis”. Urban Education 42(1):82-110.
KANE, C. J., D.P. Lubeck, S.J. Knight, M. Spitalny, T.M. Downs, G.D. Grossfeld, and P.R. Carroll. 2003. “Impact of patient educational level on treatment for patients with prostate
cancer: data from CaPSURE”. Urology 62(6):1035-1039.
KHATTAB, N. 2015. “Students’ aspirations, expectations and school achievement: what really matters?” British Educational Research Journal 41(5):731-748.
KIM, E., Hogge, I., and C. Salvisberg. 2014. “Effects of self-esteem and ethnic identity: Acculturative stress and psychological well-being among Mexican immigrants”. Hispanic Journal of Behavioral Sciences 36(2):144-163.
KIVISTO, P., and P.R. Croll. 2012. Race and ethnicity: the basics. New York: Routledge.
KLEIN, J. T. 2000. “A Conceptual Vocabulary of Interdisciplinary Science”. In: P. Weingart and N. Stehr, eds., Practising Interdisciplinarity. Toronto: University of Toronto Press.
KRUEGER, P. M., M.K. Tran, R.A. Hummer, and V.W. Chan. 2015. “Mortality attributable to low levels of education in the United States”. PloS one 10(7):e0131809.
LADSON-BILLINGS, G., and W.F. Tate. 1995. “Toward a Critical Race Theory of Education. In. A.D. Dixson, C.K. Rousseau Anderson and J.K. Donnor, eds., Critical Race Theory in Education: All God’s Children Got a Song”. New York: Taylor & Francis.
LAURITZEN, S. M., and T.S. Nodeland. 2018. “’What is the problem represented to be?’ Two decades of research on Roma and education in Europe”. Educational Research Review 24:148-169.
LI, Y., Devine, F., and A. 2008. Heath Equality group inequalities in education, employment and earnings: A research review and analysis of trends over time. Manchester: Equality and Human Rights Commission.
LIN, S. S. and J.L. Kelsey. 2000. “Use of race and ethnicity in epidemiologic research: concepts, methodological issues, and suggestions for research”. Epidemiological Review 22:187-202.
MATEOS, P., A. Singleton, and P. Longley. 2009. “Uncertainty in the Analysis of Ethnicity Classifications: Issues of Extent and Aggregation of Ethnic Groups”. Journal of Ethnic and Migration Studies 35(9):1437-1460.
MCGUINN, P. 2006. “From no child left behind to the every student succeeds act: Federalism and the education legacy of the Obama administration”. Publius: The Journal of Federalism 46(3):392-415.
MIRRA, N., Coffey, J., and A. Englander. 2018. “Warrior Scholars & Bridge Builders: Civic Dreaming in ELA Classrooms”. Journal of Literacy Research 50(4):423-445.
MONGEON, P., and A. Paul-Hus. 2016. “The journal coverage of Web of Science and Scopus: a comparative analysis”. Scientometrics, 106(1), 213-228.
MUNNIKSMA, A., P. Scheepers, T.H. Stark, and J. Tolsma. 2017. “The impact of adolescents‘ classroom and neighborhood ethnic diversity on same‐and cross‐ethnic friendships within classrooms”. Journal of Research on Adolescence 27(1):20-33.
NAZROO, J. Y. 2003. “The structuring of ethnic inequalities in health: economic position, racial discrimination, and racism”. American Journal of Public Health 93(2):277-284.
NGO, B. and S.J. Lee. 2007. “Complicating the Image of Model Minority Success: A Review of Southeast Asian American Education”. Review of Educational Research 77(4):415-453.
OGBU, J. U. 2008. Minority status, oppositional culture, & schooling. New York/London: Routledge.
PAIS, P. S. M., L.B. de Mattos, and E.C. 2018. “Teixeira. Interstate migration and human capital formation in Brazil”. International Journal of Social Economics 45(8):1159-1173.
PHINNEY, J. S. 1993. A three-stage model of ethnic identity development in adolescence. Ethnic identity: Formation and transmission among Hispanics and other minorities, In: M.E. Bernal and G. P. Knight, eds., Ethnic Identity: Formation and Transmission among Hispanics and Other Minorities, New York: State University of New York Press.
PHINNEY, J. S., G. Horenczyk, K. Liebkind, and
P. Vedder. 2001. “Ethnic identity, immigration, and well‐being: An interactional perspective”. Journal of Social Issues 57(3):493-510.
PINES, J. M., and K. 2006. Buford. “Predictors of frequent emergency department utilization in Southeastern Pennsylvania”. Journal of Asthma 43(3):219-223.
PORTER, M.F. 2001. “Snowball: A language for stemming algorithms”. http://snowball.tartarus.org/texts/introduction.html.
PORTES, P. R. 1996. Ethnicity and culture in educational psychology. Handbook of educational psychology (pp. 331-357), edited by Berliner, D.C. & R.C. Calfee, New York: MacMillan.
QUIAO, N., and T.M. Bell. 2017. “Indigenous Adolescents’ suicidal behaviors and risk factors: evidence from the National Youth Risk Behavior Survey”. Journal of Immigrant and Minority Health 19(3):590-597.
RASMUSSEN, C. 2017. “Creating segregation in the era of integration: school consolidation and local control in New Brunswick, New Jersey, 1965-1976”. History of Education Quarterly 57(4):480-514.
RALEY, R. K., M.L. Frisco, and E. Wildsmith. 2005. “Maternal cohabitation and educational success”. Sociology of Education 78(2):144-164.
ROBERTS, R. A., L.A. Bell, and B. Murphy. 2008. “Flipping the script: Analyzing youth talk about race and racism”. Anthropology & Education Quarterly 39(3):334-354.
ROBERTS, M. E., B.M. Stewart, D. Tingley, C. Lucas, J. Leder‐Luis, S.K. Gadarian, B. Albertson, and D.G. Rand. 2014. “Structural topic models for open-ended survey responses”. American Journal of Political Science 58(4):1064-1082.
SHELDON, T. A., and H. Parker. 1992. “Race and ethnicity in health research”. Journal of Public Health 14(2),104-110.
SHENG, H., and Z. Gao. 2012. “Ethnicity differences in pedometer-based physical activity levels among adolescent girls”. Journal of Exercise Science & Fitness 10(1),38-43.
SHETGIRI, R., S. Kataoka, H. Lin, and G. Flores. 2011. “A randomized, controlled trial of a school-based intervention to reduce violence and substance use in predominantly Latino high school students”. Journal of the National Medical Association.103(9-10):932-940.
SHIRLEY, E. L., and D.G. Cornell. 2012. “The contribution of student perceptions of school climate to understanding the disproportionate punishment of African American students in a middle school”. School Psychology International 33(2):115-134.
SPERA, C., K.R. Wentzel, and H.C. Matto. 2009. “Parental aspirations for their children’s educational attainment: Relations to ethnicity, parental education, children’s academic performance, and parental perceptions of school climate”. Journal of Youth and Adolescence 38(8):1140-1152.
STARFIELD, B., A.W. Riley, W.P. Witt, and J. Robertson. 2002. “Social class gradients in health during adolescence”. Journal of Epidemiology & Community Health 56(5):354-361.
STEVENS, P. A. J., and G.A. Dworkin. 2019. The Palgrave Handbook of Race and Ethnic Inequalities in Education. London: Palgrave.
STEVENS, J., M.W. Plankey, D.F. Williamson, M.J. Thun, P.F. Rust, Y. Palesch, and P.M. O‘Neil. 1998. “The body mass index‐mortality relationship in white and African American women”. Obesity Research 6(4):268-277.
VAN DEN BOOGAART, K. G., and R. Tolosana-Delgado. 2013. Analyzing compositional data with R. Berlin: Springer.
VINER, R. M., E.M. Ozer, S. Denny, M. Marmot, M. Resnick, A. Fatusi, and C. Currie. 2012. “Adolescence and the social determinants of health”. The Lancet 379(9826):1641-1652.
WALKER, L., S. Merry, P.D Watson, E. Robinson, S. Crengle, and D. Schaaf. 2005. “The Reynolds adolescent depression scale in New Zealand adolescents”. Australian & New Zealand Journal of Psychiatry 39(3):136-140.
WORRELL, F. C., M.W. Watkins, and T.E. Hall. 2008. “Reliability and validity of self-concept scores in secondary school students in Trinidad and Tobago”. School Psychology International 29(4):466-480.
WARIKOO, N. and P. Carter. 2009. “Cultural Explanations for Racial and Ethnic Stratification in Academic Achievement: A Call for a New and Improved Theory”. Review of Educational Research 79(1):366-394.
WOJKIEWICZ, R. A., and K.M. Donato. 1995. « Hispanic educational attainment: The effects of family background and nativity”. Social Forces 74(2):559-574.
YI, H., H. Zhang, X. Ma, L. Zhang, X. Wang, L. Jin, and S. Rozelle. 2015. “Impact of free glasses and a teacher incentive on children‘s use of eyeglasses: a cluster-randomized controlled trial”. American Journal of Ophthalmology 160(5):889-896.
ZAGEFKA, H. 2009. “The concept of ethnicity in social psychological research: Definitional issues”. International Journal of Intercultural Relations 33(3):228-241.
ZHU, J, and W. Liu. 2020. “A tale of two databases: the use of Web of Science and Scopus in academic papers”. Scientometrics 123(1):321-35.
New Diversities • Volume 23, No. 1, 2021
Thinking Beyond Boundaries: Researching Ethnoheterogenesis in Contexts of Diversities and Social Change
Guest Editors: Nina Clara Tiesler, Mathias Bös and Deborah Sielert (Leibniz University of Hannover)
- ISSN-Print 2199-8108
- ISSN-Internet 2199-8116